Finding Integrated Imaging Biomarker for Cancer
AI-based Radio-Patho-Genomics for Precision Medicine
- Biomarkers as major prognostic factors such as immuno-chemotherapy were generally identified from genome or pathological results, but recent studies to find prognostic biomarkers within medical images (MR/CT, PET, etc.) are ongoing.
- The advantages of medical image analysis are as follows:
1) Cross-sectional analysis is possible, not sampling-based analysis.
2) Intra/peri-tumoral analysis is possible extensively.
3) It contains objective information based on specific physical quantities. (Example: electron density in CT) - Analysis using medical images enables extracting information on the entire tumor area in a cross-sectional way which provides an opportunity to quantitatively evaluate tumor heterogeneity, one of the main characteristics of malignant tumors.
- The radiomic feature that analyzes the texture of the tumor defines intra-tumor heterogeneity by quantifying the image pattern based on the spatial relationship between pixels of a specific intensity or co-occurrence of pixel values.
- This makes it possible to derive and quantify an imaging phenotype close to a specific disease phenotype.
- Thus, It is expected that more detailed patient risk stratification will be possible through multi-omics analysis incorporating biomarkers of each level.
Finding Integrated Imaging Biomarker for Cardiac Diseases
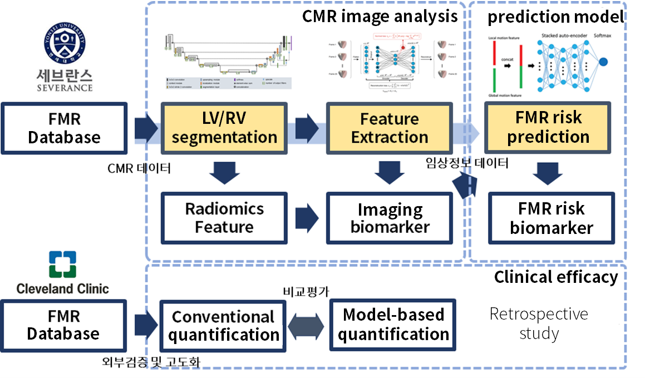
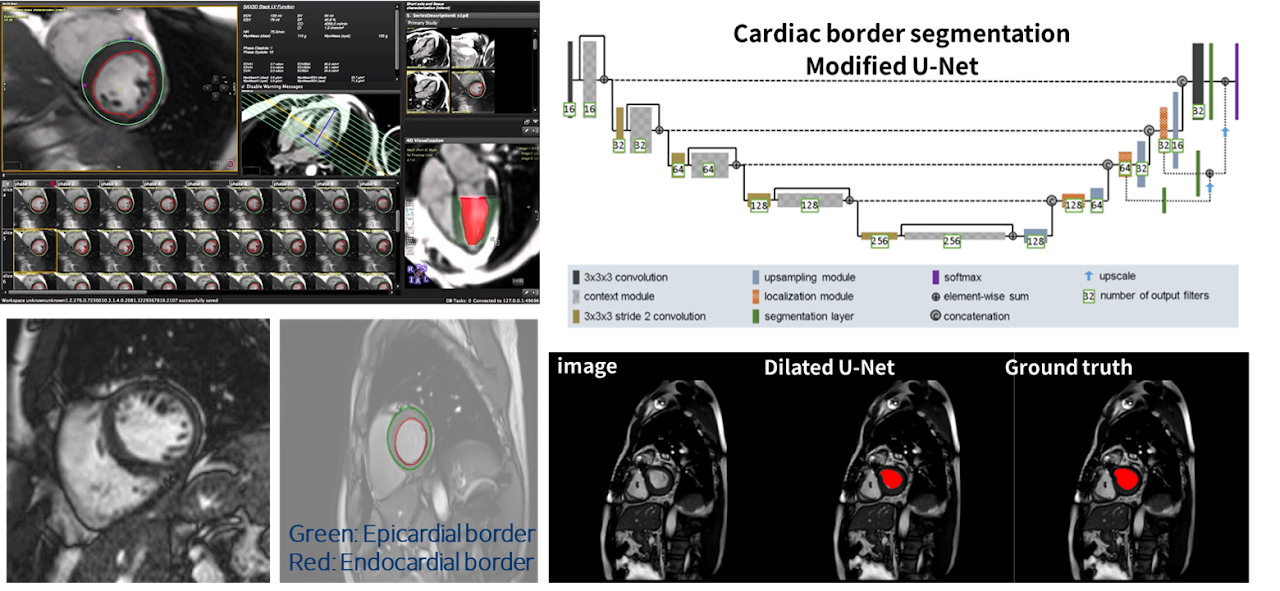
AI-based Cardiac Failure Risk Prediction with Cardiac CT/MRI
- Cardiac MR Image (CMR) is cross-sectional and objective image phenotype information for the entire heart area, so it is possible to quantitatively analyze left ventricle (LV) remodeling and myocardial fibrosis.
- CMR contains information on the movement of the heart between the systolic-diastolic phases, and by analyzing it, functional analysis of cardiac images such as the ejection fraction or cardiac output is possible.
- CMR provides the complex interplay between MV/LV functional (motion, systolic function and strain in CINE sequence), structural (analysis of mitral valve (MV) apparatus and LV abnormal findings and MV copatation depth, tenting area, LVSV, etc.), tissue (LV remodeling causes in myocardium change according to-LGE quantification or T1 value in LGE or T1 map sequence) abnormalities.
- Thus, it is expected that an imaging biomarker that has been difficult to analyze can be derived through AI-based CMR analysis.
- By deriving CMR-based imaging biomarkers of patients, it is expected that the effects of LV remodeling and infarction on treatment response or long-term prognosis and direct cardiac function can be newly defined.
Finding Integrated Imaging Biomarker for Infectious Disease
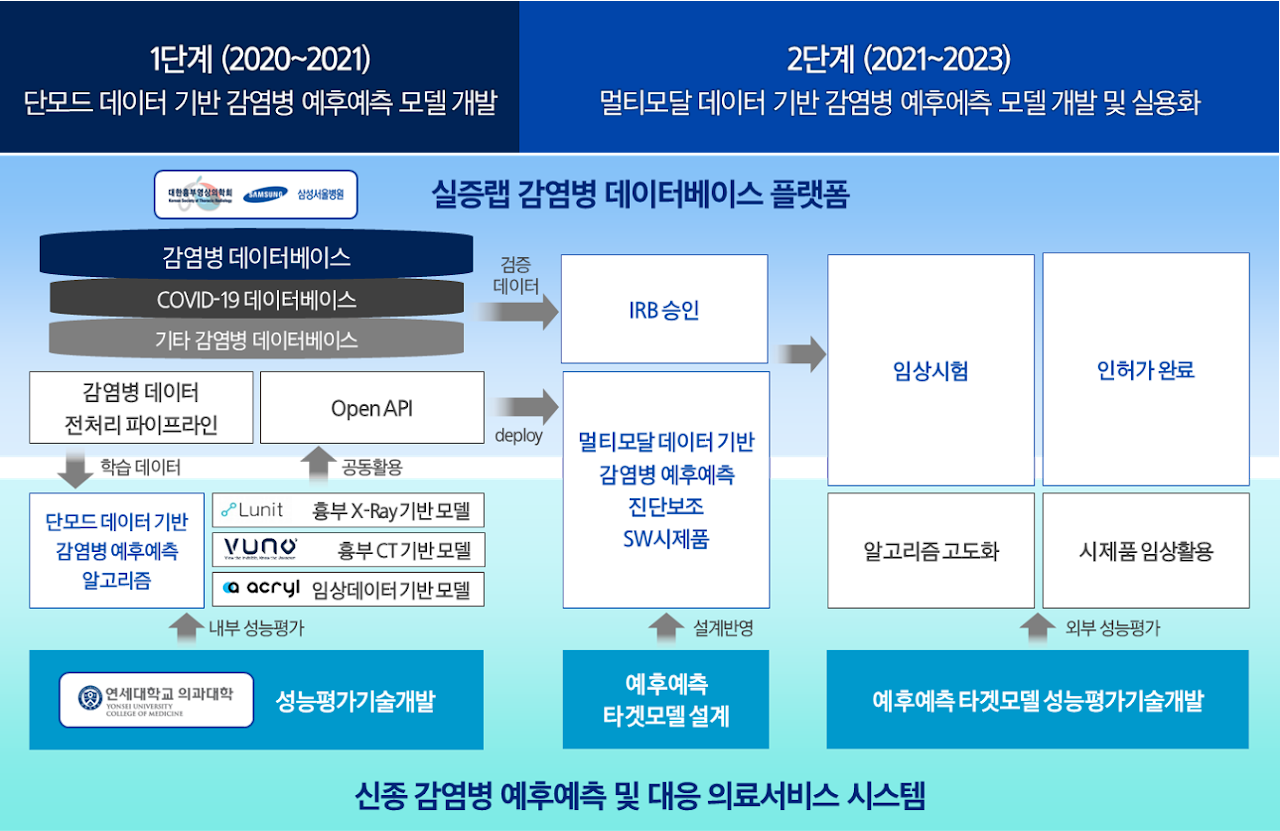
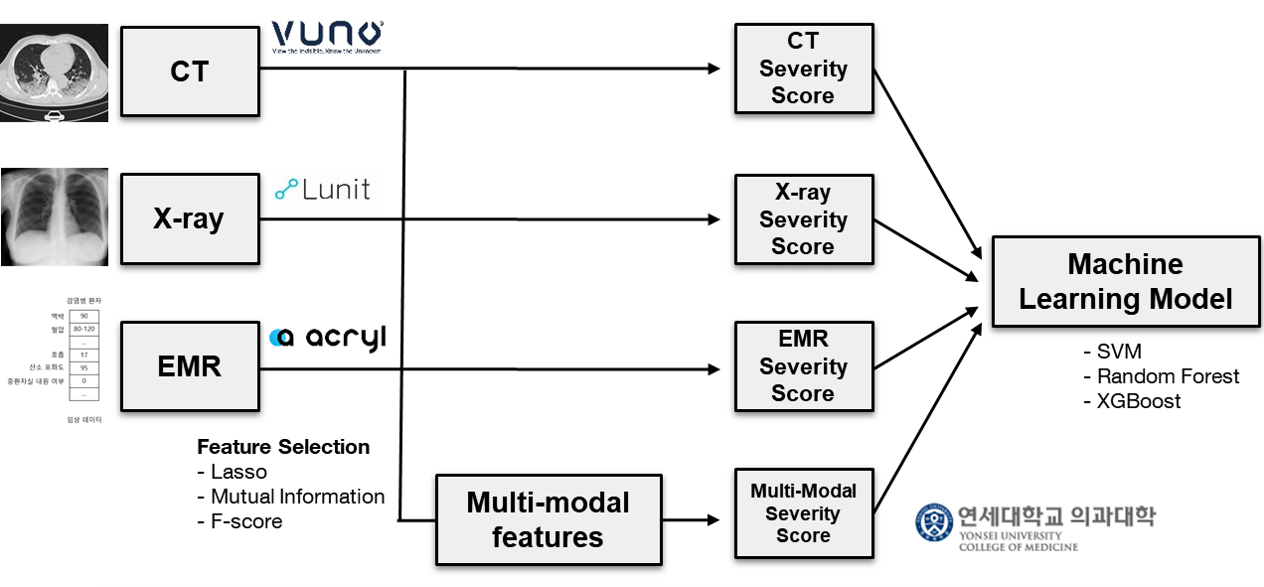
AI-based Prognostic Solution for Infectious Diseases
- During the COVID-19 pandemic, when a new infectious disease spreads, it is important to detect the disease early and implement appropriate control policy to minimize damage.
- It is expected to be able to quickly diagnose infectious diseases and predict the prognosis of patients with infectious diseases in the early stages of infection by fusion technologies such as artificial intelligence and big data.
- AI technology can comprehensively analyze/learn a specific pattern of infectious disease images of various patients. This enables the artificial intelligence to efficiently discern the subtle changes in the ROI where infectious diseases are suspected in the image.
- For initial response to infectious diseases in local governments/hospitals, AI technology to assist epidemiological investigations of infectious diseases can be developed to increase the ability to respond to infectious diseases early.
- By applying an infectious disease prognosis prediction solution using AI in clinical practice, it improves the efficiency of distribution of medical resources by predicting patients at risk of severity/death, and lowers the disease transmission rate and mortality rate of infectious disease patients by providing customized medical services.
Basic AI/ML Research for Trustworthy Medical AI system
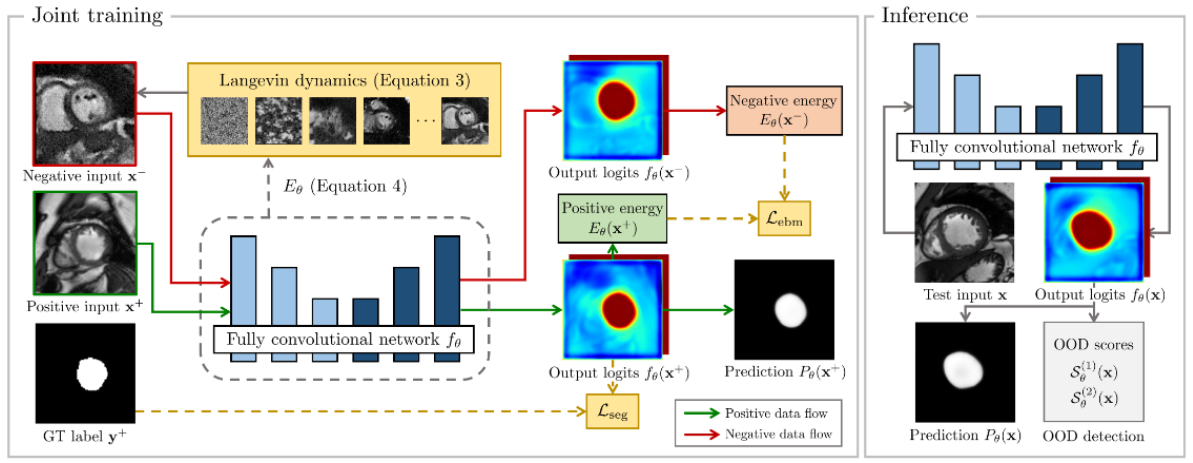
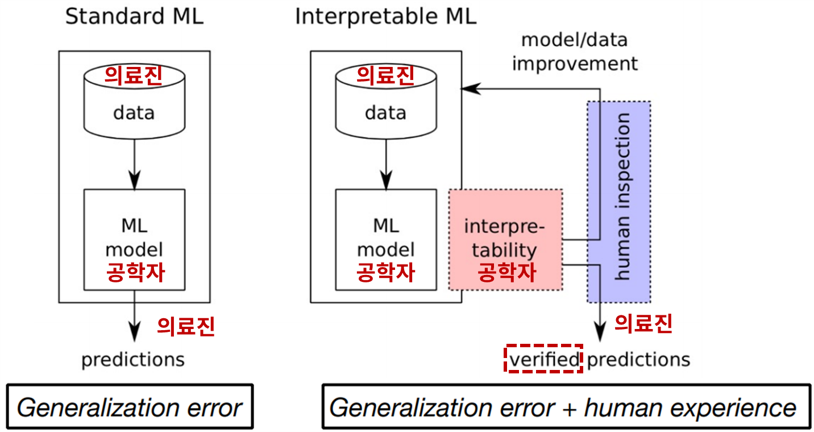
Reliable Medical AI Systems to Apply in Clinical Practice
- Various research results have been published that apply artificial intelligence technology to medical problems such as automatic of medical images in limited purposes.
- However, many researchers point to the development of a reliable medical artificial intelligence model as a major prerequisite for applying AI technology to real clinic.
- AI as an auxiliary tool for clinical decision-making can help the medical staff to make decisions for diagnosis or treatment of patients only by securing the reliability of the results.
- The methodologies/topics to deal with the technical limitations of AI models that are obstacles to securing such reliability:
1) Explainable/Interpretable AI
2) (Self) Attention Model/Causal Learning
3) Uncertainty Quantification for Model Robustness
4) Fairness
Standardization of AI-based
Medical Devices and Medical Big Data
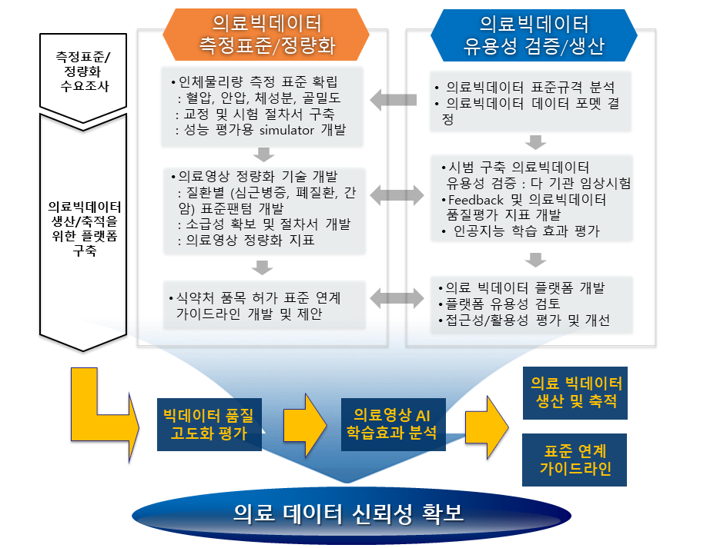
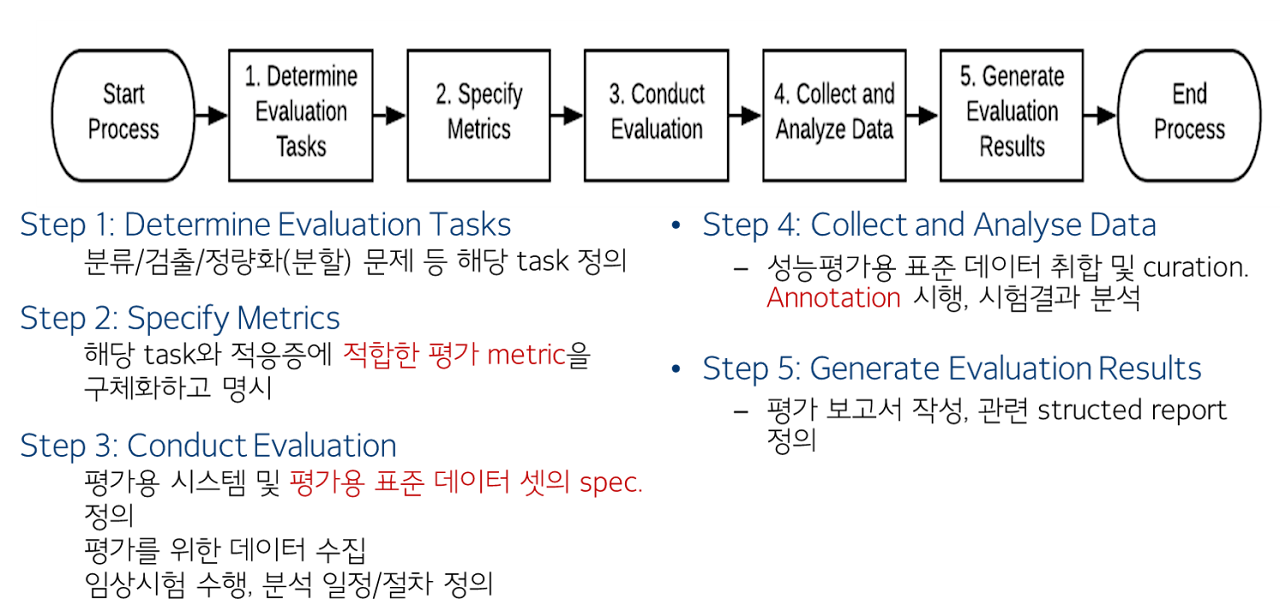
Standard Evaluation Technologies for Quality Advancement of Clinical Data
- For effective use of medical big data, reliability and interoperability of medical data must be secured.
- However, research on reliability and compatibility of data is limited, due to the diversity of mechanical performance and processing techniques of measuring devices.
- Based on measurement standards, our research team intend to establish a standardized full-cycle infrastructure or platform that reflects the entire medical workflow from data acquisition, measurement, evaluation, storage, and utilization and to establish high-quality medical big data-based technology by evaluating its effectiveness.
Standard for Performance Evaluation Technology of AI-based Medical Devices
- Development of international standards and reference data for data labeling and performance evaluation methods for various AI-based medical devices for diagnosis/prediction of diseases.
1) International standard development of AI-based medical device (AI-MD) performance evaluation method
2) Development of international data standards for the performance evaluation of AI-MD
3) Development of reference data for performance evaluation of AI-MD
4) Implementation and verification of labeling tool SW module supporting data standards